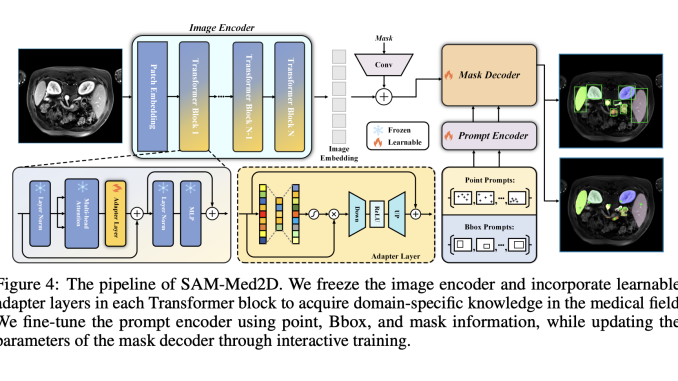
By recognizing and separating different tissues, organs, or regions of interest, medical image segmentation is essential to studying medical pictures. For more exact diagnosis and therapy, clinicians can use accurate segmentation to help them locate and accurately pinpoint disease regions. Additionally, thorough insights into the morphology, structure, and function of various tissues or organs are provided through quantitative and qualitative analysis of medical pictures, enabling the study of illness. Due to the peculiarities of medical imaging, such as its wide variety of modalities, complicated tissue and organ architecture, and absence of annotated data, most existing approaches are restricted to certain modalities, organs, or pathologies.
Because of this restriction, algorithms are difficult to generalize and modify for use in various clinical contexts. The push towards large-scale models has recently generated excitement among the AI community. The development of general AI models like ChatGPT2, ERNIE Bot 3, DINO, SegGPT, and SAM makes employing a single model for various tasks possible. With SAM, the most recent large-scale vision model, users may create masks for certain regions of interest by interactively clicking, drawing bounding boxes, or using verbal cues. Significant attention has been paid to its zero-shot and few-shot capabilities on natural photos across various fields.
Some efforts have also concentrated on the SAMs’ zero-shot capability in the context of medical imaging. However, SAM finds it difficult to generalize to multi-modal and multi-object medical datasets, leading to variable segmentation performance across datasets. This is because there is a considerable domain gap between natural and medical images. The cause can be linked to the methods used to gather the data: due to their specific clinical purpose, medical pictures are obtained using particular protocols and scanners and displayed as various modalities (electrons, lasers, X-rays, ultrasound, nuclear physics, and magnetic resonance). As a result, these images deviate substantially from real images since they depend on various physics-based features and energy sources.
Natural and medical images differ significantly in terms of pixel intensity, color, texture, and other distribution features, as seen in Figure 1. Because SAM is trained on only natural photos, it needs more specialized information regarding medical imaging, so it cannot be immediately applied to the medical sector. Providing SAM with medical information is challenging due to the high annotation cost and inconsistent annotation quality. Medical data preparation needs subject expertise, and the quality of this data differs greatly between institutions and clinical trials. The amount of medical and natural images varies significantly due to these difficulties.
The bar chart in Figure 1 compares the data volume of publicly available natural image datasets and medical image datasets. For instance, Totalsegmentor, the largest public segmentation dataset in the medical domain, also has a significant gap compared to Open Image v6 and SA-1B. In this study, their objective is to transfer SAM from natural images to medical images. This will provide benchmark models and evaluation frameworks for researchers in medical image analysis to explore and enhance. To achieve this goal, researchers from Sichuan University and Shanghai AI Laboratory proposed SAM-Med2D, the most comprehensive study on applying SAM to medical 2D images.
Check out the Paper and Github. All Credit For This Research Goes To the Researchers on This Project. Also, don’t forget to join our 29k+ ML SubReddit, 40k+ Facebook Community, Discord Channel, and Email Newsletter, where we share the latest AI research news, cool AI projects, and more.
If you like our work, you will love our newsletter..
Aneesh Tickoo is a consulting intern at MarktechPost. He is currently pursuing his undergraduate degree in Data Science and Artificial Intelligence from the Indian Institute of Technology(IIT), Bhilai. He spends most of his time working on projects aimed at harnessing the power of machine learning. His research interest is image processing and is passionate about building solutions around it. He loves to connect with people and collaborate on interesting projects.
Be the first to comment